MIT researchers have developed a way of doing machine learning that enables semantically related concepts to reinforce each other and improving image classification during training by giving the system partial credit when it only gets part of a semantic sequence correct.
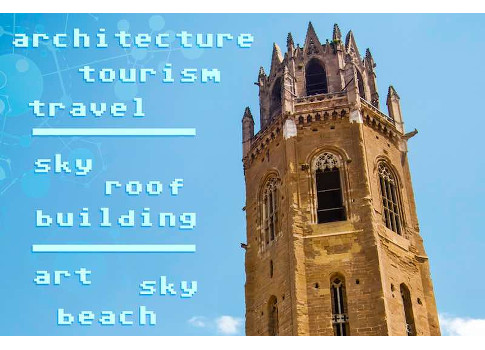
Machine learning, which is the basis for most commercial artificial-intelligence systems, is intrinsically probabilistic. An object-recognition algorithm asked to classify a particular image, for instance, might conclude that it has a 60% chance of depicting a dog, but a 30% chance of depicting a cat.
At the Annual Conference on Neural Information Processing Systems in December, MIT researchers will present a new way of doing machine learning that enables semantically related concepts to reinforce each other. So, for instance, an object-recognition algorithm would learn to weigh the co-occurrence of the classifications "dog" and "Chihuahua" more heavily than it would the co-occurrence of "dog" and "cat."
In experiments, the researchers found that a machine-learning algorithm that used their training strategy did a better job of predicting the tags that human users applied to images on the Flickr website than it did when it used a conventional training strategy.
"When you have a lot of possible categories, the conventional way of dealing with it is that, when you want to learn a model for each one of those categories, you use only data associated with that category," said Chiyuan Zhang, an MIT graduate student in electrical engineering and computer science and one of the new paper’s lead authors. "It’s treating all other categories equally unfavorably. Because there are actually semantic similarities between those categories, we develop a way of making use of that semantic similarity to sort of borrow data from close categories to train the model."
Zhang is joined on the paper by his thesis advisor, Tomaso Poggio, the Eugene McDermott Professor in the Brain Sciences and Human Behavior, and by his fellow first author Charlie Frogner, also a graduate student in Poggio’s group. Hossein Mobahi, a postdoc in the Computer Science and Artificial Intelligence Laboratory, and Mauricio Araya-Polo, a researcher with Shell Oil, round out the paper’s co-authors.
Close counts
To quantify the notion of semantic similarity, the researchers wrote an algorithm that combed through Flickr images identifying tags that tended to co-occur – for instance, "sunshine," "water," and "reflection." The semantic similarity of two words was a function of how frequently they co-occurred.
Ordinarily, a machine-learning algorithm being trained to predict Flickr tags would try to identify visual features that consistently corresponded to particular tags. During training, it would be credited with every tag it got right but penalized for failed predictions.
The MIT researchers’ system essentially gives the algorithm partial credit for incorrect tags that are semantically related to the correct tags. Say, for instance, that a waterscape was tagged, among other things, "water," "boat," and "sunshine." With conventional machine learning, a system that tagged that image "water," "boat," "summer" would get no more credit than one that tagged it "water," "boat," "rhinoceros." With the researchers’ system, it would, and the credit would be a function of the likelihood that the tags "summer" and "sunshine" co-occur in the Flickr database.
The problem is that assigning partial credit involves much more complicated calculations than simply scoring predictions as true or false. How, for instance, does a system that gets none of the tags completely right—say, "lake," "sail," and "summer"—compare to one that makes only one enormous error—say, "water," "boat," and "rhinoceros"?
To perform this type of complicated evaluation, the researchers use a metric called the Wasserstein distance, which is a way of comparing probability distributions. That would have been prohibitively time-consuming even two years ago, but in 2014, Marco Cuturi of the University of Kyoto and Arnaud Doucet of Oxford University proposed a new algorithm for calculating the Wasserstein distance more efficiently. The MIT researchers believe that their paper is the first to use the Wasserstein distance as an error metric in supervised machine learning, where the system’s performance is gauged against human annotations.
Human error
In experiments, the researchers’ system outperformed a conventional machine-learning system even when the criterion of success was simply predicting the tags that Flickr users had applied to a given image. But the difference was even more acute when the criterion of success was the prediction of tags that were semantically similar to those applied by Flickr users.
That may sound circular: A system that factors in semantic similarity is better at predicting semantic similarity. But when a Web user is trying to find images online, a general thematic correspondence may well be more important than a precise intersection of keywords.
Moreover, the tags that users assign to any given Flickr image can be a motley assortment. Automatically generated tags clustered according to semantic similarity could be more useful than those applied by humans. One image in the researchers’ test set, for instance, depicted a uniformed mountain biker wearing a crash helmet biking down a hilly trail. The actual tags were "spring," "race," and "training." But the trees in the image are bare, the grass is brown, and the tags "race" and "training" can’t both be right. The researchers’ system came up with "road," "bike," and "trail"; the conventional machine-learning algorithm produced "dog," "surf," and "bike."
Finally, if some other measure of the notion of semantic similarity proved better able to capture human intuition than co-occurrence of Flickr tags, then the MIT researchers’ system could simply adopt it instead. Indeed, a longstanding and ongoing project in artificial-intelligence research is the assembly of "ontologies" that relate classification terms hierarchically—dogs are animals, collies are dogs, Lassie was a collie. In future work, the researchers hope to test their system using ontologies standard in machine-vision research.
Massachusetts Institute of Technology (MIT)
– Edited by Chris Vavra, production editor, Control Engineering, CFE Media, [email protected].
ONLINE extra
See additional stories about machine design and machine learning below.