Future automation safety will involve machines that learn. Artificial intelligence (AI) and machine learning (ML) advances can prompt robots and other industrial machinery to learn from a massive pool of safety-related data to make today’s manufacturing applications safer than they’ve ever been.
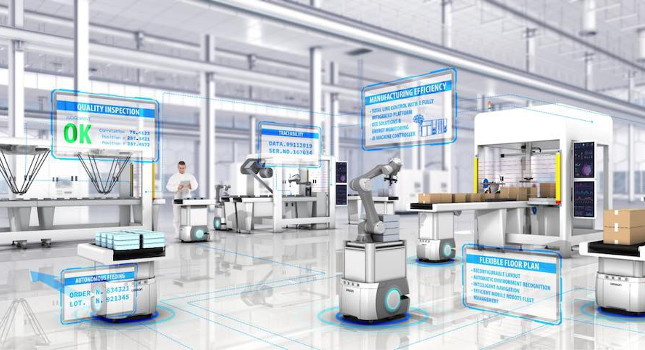
The primary focus of industrial safety measures used to involve isolating the machines from personnel as much as possible. Nowadays, technological advancements in automation equipment have made it possible for machines — particularly collaborative robots — to work with human operators in close quarters. These technologies have features like rounded edges and force-feedback sensors to reduce the likelihood of an injury upon contact with an operator.
In addition to these developments, automation systems are in the process of transitioning from fixed to autonomous mobility. One manufacturing solution poised to transform manufacturing involves connecting a collaborative robotic arm to a self-navigating mobile base. For control design engineers to employ risk reduction measures for innovations like this, they need to understand the requirements of the technology, its potential hazards, and the ways operators will be working with it.
As systems get more complex, it becomes ever more challenging for manufacturers to analyze all the data applicable to a risk reduction plan. The amount of information can be overwhelming, and the mechanisms available to govern the decision-making process are limited. Artificial intelligence (AI) can help overcome these limits by providing multiple options the automation designer may not have considered, and this quality combined with its sheer number-crunching power makes it an essential component of today’s automation systems.
Determining the safety requirements of near-future technology
The standard IEC 62061, which deals with safety-related electrical, electronic and programmable electronic control systems, defines functional safety as:
“Part of the safety of the machine and the machine control system which depends on the correct functioning of the SRECS (safety-related electrical control system), other technology safety-related systems and external risk reduction failures.”
This definition makes more sense when re-framed as a goal, which is to design a system that, in the event of a failure, will fail in a predictable manner. The manufacturing industry has become proficient with hardware solutions. Safety standards provide manufacturers, integrators, and end users with a best-practice methodology to achieve tolerable risk levels for these solutions. We can also draw upon these standards to help determine the safety requirements for developing technologies.
At present, there are no safety standards specifically for an industrial robot integrated with a mobile platform. Nonetheless, we can gather relevant information from existing safety standards, such as ANSI B11.0 or ISO 12100 for risk assessments, ANSI RIA R15.06 or ISO 10218-2 for industrial robotic systems, ANSI/RIA R15.606 or ISO 15066 for collaborative robots, ANSI/IT SDF B56.5 or EN 1525 (to be replaced by ISO 3691-4) for industrial trucks, and ISO 13849-1 for failure prediction and validation. Sources of hazards and recommended risk reduction measures should be available in vendor manuals.
After determining the applicable standards, the engineer needs to evaluate and design for things that influence the space, such as workflow, obstacles, accessibility, misuse and training. Technology also plays a role, as feedback error can cause measurement noise that affects position tracking, and compliance in the joints can have inherently nondeterministic behaviors. The engineers also should consider ways in which the system absorbs energy, the methods used to limit forces, and the use of safety functions.
Where artificial intelligence comes into play
The main challenge of ensuring the safety of near-future technologies is not a lack of applicable information, but rather an overabundance of it. When there are too many variables, the major limitations of developing binary rules to represent past experiences become more apparent. As technology advances while the development of standards lags, designers are often left with making future predictions. This causes them to overestimate or underestimate the necessary safety functions.
Manufacturers can deal with this information more effectively if they expand their toolset supporting the data-crunching and decision-making processes. In particular, they can find a welcome solution in the form of AI and machine learning (ML) algorithms. An AI system could recommend new system-specific guidelines based on case studies and research data as these become available.
ML brings hidden correlations to light by analyzing large amounts of data to discover underlying patterns and trends that aren’t readily visible using traditional statistical tools. Humans can find abstract models from these correlations and perform experiments to determine how well the models work. Designers and engineers can rely on a smart system to guide them through a design, making sure to incorporate the best approaches and stay as close as possible to the desired solution.
AI is important for eliminating biases that could hamper decision-making. Since memory is a large part of how the brain makes decisions, experts’ perceptions of past experiences can create biases that affect how they deal with new situations. An expert also could either fail to recognize that crucial information is missing or make the mistake of starting with an endpoint solution at the beginning of the decision-making process. ML algorithms reduce biases because they find patterns in the current and actual data that help solve a particular problem as part of the process, using either a supervised training set or an unsupervised starting point.
From hardware-driven safety systems to software-based solutions that use AI
Since the majority of today’s safety systems are separated from rest of the system, they have a reactive response to hazardous occurrences. The levels and types of risk change when the system changes or wears down, or if it includes mobile technologies such as self-navigating robots. For example, drift may occur on a robot as it heats up and the bearings wear down; changing an end-effector can alter a robot’s potential for harm. Other changes may occur from variations in the characteristics of incoming materials or the effects of ambient conditions such as temperature and vibration.
As automation transitions from hardware to solid-state and software-driven systems, data scientists and programmers are using AI models to determine what is possible while subject matter experts determine what is usable. An example of a practical AI application is one that tracks and analyzes everything within the manufacturing process to find possible issues before they emerge. It also could monitor the ambient conditions to analyze their impact on a machine’s functionality, and then use the discovered correlations to make predictions for the future and optimize the process of scheduling repairs.
AI-monitored predictive maintenance is essential for reducing the likelihood of hazardous events and improving the decision-making process in case such events occur. Unexpected equipment shutdowns can create stressful situations in which people make quick, error-prone decisions based on gut reactions rather than rational decision-making skills. By continuously monitoring the system to detect changes that might be indicative of a problem, the ML algorithms gives human operators a heads-up that something’s wrong, and the advance notice will make it easier for the operators to make controlled decisions.
This is important given the current transition of manufacturing from a static enterprise to a largely mobile one. Robots were once designed to perform the same task for well over a decade. With today’s demand for production lines that are flexible and adaptable, robots stay on the same task for two years or less. Some will be attached to mobile bases that zip across the factory floor of their own accord. This means the safety system needs to be updated much more frequently, and the number of variables to consider is exponentially larger than before. Only ML can successfully deal with all these variables.
Artificial intelligence also can help make robots and other equipment more ergonomic – a safety concern in its own right. Robots are designed to function with a person regardless of height, while the human is often the entity responsible for any necessary adaptation. Built-in ML algorithms ideally allow the robot itself to learn how best to work with its operator to provide maximum comfort. Based on cues taken from the operator, the robot could discover whether it’s been paired with a right-handed or left-handed person, or if the operator has some sort of physical disability.
Data-driven challenges
The driving force behind the introduction of AI into industrial equipment is the sheer plethora of safety-related information in today’s manufacturing facilities. There’s so much data out there even experienced workers are having trouble learning and retaining it all — not to mention the junior employees who are just stepping into their first manufacturing job. By getting the machines to learn for themselves, companies can take advantage of a powerful risk-reduction tool that will offer both short-term and long-term data on safety requirements in a changing environment.
Everything being applied in safety solutions today is based on things engineers, operators and manufacturers have learned from the past. In that sense, AI isn’t all that different. Neither humans nor algorithms start off knowing anything about industrial safety — we must all make connections using bits and pieces from past experiences that we can apply to new situations. We learn what works and what doesn’t work, and we use this knowledge to make future decisions. AI works the same way.
Tina Hull is TUV Functional Safety Expert and product engineer, Omron Automation Americas. Edited by Chris Vavra, production editor, Control Engineering, CFE Media, [email protected].
MORE ANSWERS
Keywords: Machine safety, artificial intelligence, machine learning
Artificial intelligence (AI) can help manufacturers analyze the data involved with safety issues.
AI can also help make robots and other equipment more ergonomic and adapt to specific workers.
Collaborative robots, thanks to AI and machine learning (ML) advances, can be designed to interact in safer ways with humans.
CONSIDER THIS
How else can artificial intelligence and machine learning help improve your plant-floor operations?