Industrial manufacturers are using edge controllers and industrial PCs (IPCs) to implement practical analytics initiatives from the edge up, instead of from the enterprise down.
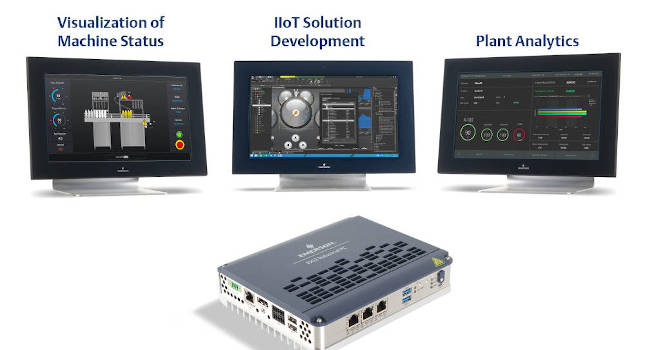
Whether a manufacturer runs a few automated machines at a single location, or a fleet of equipment across many sites, there is always a need for better visibility, data and analytics. Without access to the source data, and a way to analyze it, these companies are mostly operating based on experience.
For many reasons, manufacturers may be wary about commencing even basic analytical efforts. Their core systems are already operating and there may not be a clear understanding of the potential payback. There also can be great concern around the hardware, software and integration needed to obtain any type of meaningful analytics.
However, these companies may be surprised to find they can build analytics by starting small at the edge instead of jumping into a large effort at the enterprise. By gathering many sources of Industrial Internet of Things (IIoT) little data at the edge, and then processing it into Big Data if necessary, users can create valuable and actionable information. Edge computing hardware and software are making this possible.
Analytics: Bottom-up instead of top-down
Many manufacturers may understand analytics initiatives to be enterprise-wide endeavors, in the domain of a massive manufacturing execution system (MES). These companies may be concerned about the resources, timing, and cost required for a big information technology (IT) type project of this type, especially when their skillset and focus may be more oriented toward plant-level operational technology (OT).
Another option exists, enabled by modern edge controllers, industrial PCs (IPCs), and OT-focused software suites. End users, systems integrators, and original equipment manufacturers of all types can build practical analytics systems bottom-up from the edge of the machine, instead of top-down from the enterprise. Edge analytics can offer immediate returns such as delivering overall equipment effectiveness (OEE) metrics, and they can also provide deeper analytics with the capability for scale-up into the enterprise.
Know the machine
Shifting computational capability from the enterprise to the edge makes a lot of sense for many reasons. Most of the most relevant data is available on-board the machine itself, although smart sensors and other external systems in the area can also be incorporated. While transmitting and storing hundreds or thousands of high-fidelity data points to the enterprise level for eventual analysis is possible, it can be expensive and often impractical.
This is because when traditional automation systems are used in this role, every new sensing point signal must be manually configured with context and engineering units, and mapped through multiple communication links and systems to reach the enterprise level. Important signals can lose timeliness along the way, while unimportant signals consume bandwidth and storage.
For the most responsive results, important metrics such as the following are best processed at the edge, where the data originates (Figure 1):
- OEE (availability, performance and quality)
- Runtime hours
- Throughput and scrap
- Energy consumption
- Machine status.
Users can access the raw data and resultant calculations directly, and transmit the consolidated results up to the cloud for eventual higher-level analysis. Edge processing in this way is ideal for understanding each machine, which is a high-value initial step, and then expanding the analysis to a production line or fleet of equipment.
Because end users often operate many machines, often distributed across many sites, any machine-level IIoT solution must be scalable to the plant and then the enterprise.
Machine to plant to enterprise
Traditional programmable logic controllers (PLCs) and human-machine interfaces (HMIs) can certainly perform some edge computations and higher-level communications, but they are much more focused on providing real-time control functionality. Therefore, end users often need edge computing options with better processing capability, support for more OT and IT communication protocols, and software tailored for analytical tasks.
Edge controllers and IPCs are two approachable platforms to help users build IIoT and edge analytics into new machines or add them to existing operations. Edge controllers include PLC functionality, but they also provide a second independent but integrated on-board operating system able to execute advanced visualization, analytical and communication duties.
IPCs are full standalone computers which can perform these tasks also. Both types of edge computing platforms are specifically designed to withstand the high temperature and vibration environments where they would be installed on machines and in factory control cabinets at the edge.
What sets edge controllers and IPCs apart from standard PLCs and HMIs is their ability to run software applications and suites to act in one or more of the following roles (Figure 2):
- IIoT data connectivity to controllers, smart sensors, and condition monitoring equipment
- Communication gateway to other peer or higher-level systems
- Visualization and dashboarding of machine status and analytics
- Analytical computations for OEE and energy sustainability.
Whether the end user is an original equipment manufacturer (OEM) building a machine or technical staff at an operating plant adding IIoT, the right visualization and analytics software will speed their efforts. These users should look for software suites offering a wizard approach for the most common tasks, such as creating OEE metrics, to reduce time and cost of implementation. The software should be suitable for starting small on a local per-machine basis, but it also must provide more advanced analytics and be scalable from this granular level up to a plant- and then enterprise-wide level.
IIoT-enabled machines
Most HMI software packages connect to one or more types of PLCs, but more advanced versions can connect to other edge located sensors using protocols like EtherNet/IP, Modbus TCP, and OPC UA. HMI software that is IIoT-capable should also support the message queuing telemetry transport (MQTT) protocol for cloud connectivity. These software platforms often provide additional features such as remote and mobile connectivity.
While HMI software may include some fundamental analytical functions, it is often necessary to use complementary software packages with extended features for providing plant-level analytics and energy efficiency evaluation. This is the reason why an integrated platform delivering IIoT, local HMI, distributed HMI and supervisory control and data acquisition (SCADA), and analytics is the best approach to enable scalability (Figure 3).
Connectivity, communication, visualization, and analytical software are available individually or in a suite of products, and sometimes pre-loaded for convenience on edge controllers and IPCs. With the right hardware, HMI software, and analytical software in place, any machine can be IIoT-enabled.
Applying edge computing
Companies involved with industrial packaging and containers, and also manufacturers of a diverse mix of specialty products, have benefited from implementing OEE at their facilities. Edge computing solutions have allowed them to optimize their overall productivity by monitoring production flows in real-time and calculating the OEE, without stopping production.
These users have relied on configuration wizards, enabling them to create in minutes projects to calculate key performance indicators (KPIs), OEE and downtime. Wizards are tools providing a step-by-step procedure guiding users to import specific information and parameters needed to create an entire OEE application. Operators use dashboards to visualize production, recipes, machine information, number of units produced, total running time and downtime, and estimated versus actual cycle time. This information is kept in a database and detailed reports can be created. The results have brought great advantages for production line operators and managers that focus on achieving a set performance goals.
Some companies decide to keep their analytics local so they can make decisions at the plant level, while others require the information to be available via the cloud so it can be incorporated with other data sets or analyzed at the fleet level. An open, modular, scalable and flexible platform is ideal to implement IIoT and to deliver various deployment scenarios.
Machine IIoT from edge to enterprise
Industrial analytics may seem esoteric, and many companies may believe it is beyond their immediate grasp to implement any kind of meaningful IIoT initiatives. The cost and complexity of starting a top-down IT-centric project can be intimidating.
A new generation of edge computing hardware, networking, protocols and software has changed this thought process. Vendors with deep OT experience have introduced edge controllers and IPCs tough enough to withstand the factory floor and on-machine environment. Wired and wireless Ethernet, combined with communication protocols like OPC UA and MQTT, permit users to tap into any edge-located data source, and to send information up to higher-level systems.
These essentials let users collect many data sets at the machine, production line, manufacturing and facility levels. With the right source data available, users can employ OT-focused HMI and analytical software specifically designed to scale from a single machine up to the enterprise. This approach is a realistic way for end users to start with IIoT-enabled machines as they build up to an enterprise-wide information intelligence system.