More powerful and cost-effective computing combined with advancements in artificial intelligence (AI) are helping predictive maintenance to detect anomalies, which predicate a maintenance action when needed. Edge computing brings decision-making and intelligence as close to the process as possible.
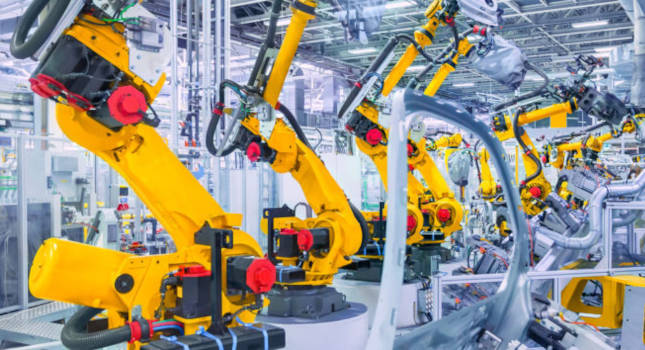
Learning Objectives
- AI is being applied to improve industrial predictive maintenance.
- AI deployments have key requirements in industrial applications.
- Link to an online course from Control Engineering and CFE Media and Technology with more on related topics.
Edge Machine Learning, Artificial Intelligence (AI) Insights
- Machine learning can be applied at the edge of applications to advance use of predictive maintenance in smart factories.
- Digital transformation is advancing as AI is applied to predictive maintenance application with help from application-specific inference engines at the edge.
What are the best ways to get started with machine learning (ML) and predictive maintenance, what are benefits and challenges, and how can strategic partnerships help? More powerful and cost-effective computing combined with advancements in artificial intelligence (AI) are bringing the next era of digital transformation in manufacturing.
AI, coupled with predictive maintenance, use parameters from the operating conditions of equipment and intelligence from application-specific inference engines at the edge to detect anomalies, which predicate a maintenance action when needed. This technology brings decision-making and intelligence as close to the process as possible. When it comes to varying tiers of maintenance strategies, reactive, preventive and predictive maintenance are all important and have their places. New tools with edge computing can enhance maintenance efficiency.
Machine learning, operating conditions, predictive maintenance
Matt Dentino, vice president, client engagement, Braintrust; and Mitsuo Baba, senior director, IoT and infrastructure business unit, Renesas Electronics Corp., speakers in the webcast, will cover the following learning objectives in the Oct. 4 webcast (archived for a year).
- Learn the benefits of machine learning and predictive maintenance
- Understand the challenges of predictive maintenance
- Explore AI and predictive maintenance for anomaly detection
- How to get started with predictive maintenance technologies.
Key requirements for AI deployments
To help with a diversity of applications, higher performance with new AI, and human interaction services, three key requirements for deploying AI applications are flexibility, power efficiency and real-time operation.
AI tools applied at the edge of applications resolve numerous challenges present in smart factories, including predictive maintenance challenges. The webcast includes many more details, examples and will answer questions at the end.
Matt Dentino is vice president, client engagement, Braintrust; and Mitsuo Baba is senior director, IoT and infrastructure business unit, at Renesas Electronics Corp. Edited by Mark T. Hoske, content manager, Control Engineering, CFE Media, [email protected].
KEYWORDS: Artificial intelligence (AI), machine learning (ML), predictive maintenance
CONSIDER THIS
Are you using AI most effectively for predictive maintenance and smart factory applications?