Neuromorphic computing, which is designed to emulate the self-organizing and self-learning nature of the brain, could help enable Big Data and artificial intelligence (AI) applications.
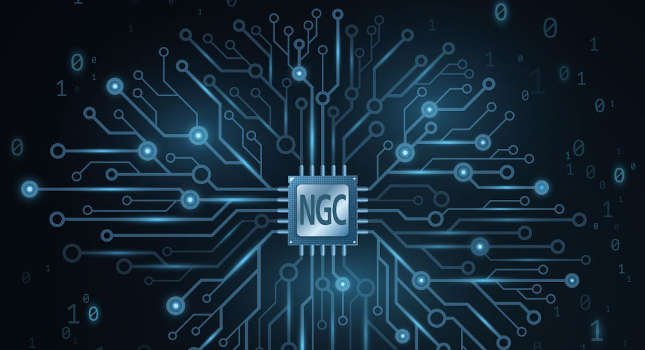
Increasing digitalization is constantly driving the demands on electronic hardware. Speed, performance, miniaturization and energy efficiency are becoming increasingly important when it comes to enabling Big Data and artificial intelligence (AI) applications. A promising solution approach is offered by so-called neuromorphic computing, which aims to emulate the self-organizing and self-learning nature of the brain.
Our hunger for data is insatiable. Ninety percent of people in Germany are online, and the trend is rising. In addition, machines, cars and technical objects are continuously sending and receiving data as a result of increasing digitalization. Forecasts predict data traffic of 172 terabits per second this year – the hourly equivalent of the data volume of all the world’s motion pictures. Managing and interpreting this gigantic volume of data increasingly requires big data applications and artificial intelligence. As a result, new types of resource- and energy-efficient computing architectures are coming into focus – especially so-called neuromorphic computing. The goal here is to emulate the functionality of the world’s most energy-efficient and flexible memory and enable a high degree of plasticity.
Advantages of neuromorphic architecture
“Classical computers perform calculations sequentially and process the data in a central memory. This means that computing power depends on the data transfer rate between processor and memory. In contrast, our brain is a multi-tasking master – it processes a large amount of data simultaneously and uses parallel neural networks. In doing so, it is extremely resource- and energy-efficient. Neuromorphic computers are trying to emulate this architecture. Crossbar architectures based on nonvolatile memories such as ferroelectric field-effect transistors are particularly promising,” said Dr. Wenke Weinreich, division director at the Center Nanoelectronic Technologies of the Fraunhofer Institute for Photonic Microsystems IPMS in Dresden.
Weinreich, along with her team, develops these non-volatile memories and integrating them into neuromorphic chips that have particularly high computing power while being extremely energy-efficient.
Fraunhofer IPMS is working on new, non-volatile memory technologies based on ferroelectric hafnium dioxide (HfO2) for analog and digital neuromorphic circuits. Ferroelectric materials are characterized by a change in their polarization when an electric field is applied. After the voltage is switched off, the polarization state is maintained. Using these ferroelectric field-effect transistors (FeFET) based on hafnium dioxide in 28 or 22 nm technology nodes, the weight values required for deep-learning algorithms can not only be stored directly in the chip, but can also be computed with (so-called in-memory computing). Similar to the human brain, the hardware architecture of the chips is designed in such a way that information is already stored in the system and is non-volatile. There is no need for complicated data transfer between the processor and the memory; computing is already performed on the chip.
In contrast to the perovskite-based materials used to date, hafniumoxide-based memories are CMOS-compatible, lead-free and scalable down to very small technology nodes. As the only non-volatile memory concept, ferroelectric memories are operated purely electrostatically and are therefore particularly power-saving, since only the transfer currents of the capacitors have to be used to write data. Memory and chip development is driven along the entire value chain, from applied materials research, device development, integration architecture and IP generation to integrated systems.
– Edited from a Fraunhofer IPMS press release by CFE Media.