Two system integration thought leaders offer advice on effective automation communication. Part 1 details effective automation through data collection. Link to other parts.
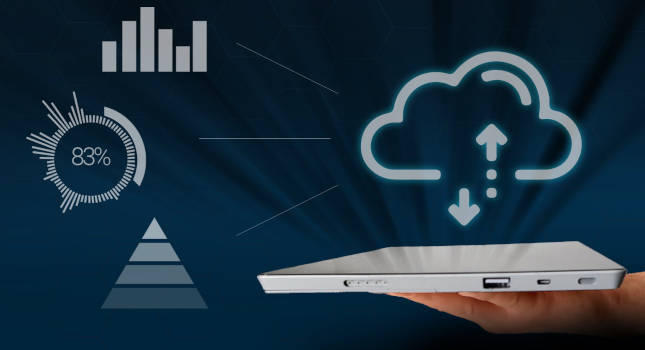
Automation application insights
- System integrators can play a key role in improving automation applications by providing their expertise from past projects to show different perspectives.
- Developing a thorough automation response based on the data collected and using that data to help inform the next course of action is the best approach.
- System integrators do face challenges because they are often the last ones on the site and have to catch up to understand the situation and find a solution that works for the unique application.
No matter the automation technologies being considered or applied, universal rules apply; a significant portion includes collecting the right information for the automation project. Whether just starting or a seasoned expert, heed this advice when applying automation. Automation can do quite a bit, but do not over-reach automation’s capabilities. Automation cannot cure overly complex operations. Get the right design first, then apply the right automation. Real-world examples will help fill skills gaps with smart manufacturing. This has been edited for clarity.
Control Engineering content manager, Mark T. Hoske, moderated the webcast “Best practices for effective automation applications.” The Aug. 16, 2022, course, archived as a webcast for a year, aims to answer the following questions about optimizing automation applications:
-
Assess the process or processes under consideration for automation.
-
Determine if a redesign is needed prior to automating.
-
Consider key ways automation can influence and enhance process design.
-
Identify steps for an automation implementation.
-
Examine lessons learned and best practices for effective automation applications.
Chris Clayton, advanced manufacturing engineer for Applied Manufacturing Technologies, and Kevin Tom, lead automation engineer at E Tech Group, offer insights on automation effectiveness through their experiences and a case study example. This has been edited for clarity.
Experts in effective automation applications
Chris Clayton is an advanced manufacturing engineer for Applied Manufacturing Technologies. He specializes in automation consulting, 3D simulation and animation and sales proposal support. He’s worked for AMT clients in a variety of industries, including aerospace and automotive. He’s also supported customers navigating the complex transition from manual processing to automation for products ranging from gelatin and pudding to fire trucks and grain trailers.
Kevin Tom is the lead automation engineer at E Tech Group. He specializes in process control system design, implementation, testing, and validation. He primarily works in the highly regulated life science industries, applying his attention to details and adherence to good manufacturing practices. He holds a degree in biochemical engineering from University of California Davis.
I’m Mark Hoske, Control Engineering, covering automation controls and instrumentation since 1994. I have a Bachelor of Science degree in journalism, and I’ve been writing and editing since 1982, and I’ve been writing about technology since 1987.
Automation is a data-driven process
Chris Clayton: I am an advanced manufacturing engineer at AMT, and one of my main roles is consulting with clients who are considering automation. These often are companies that are running a predominantly manual process and are just now dipping their toes into the automation world, so as part of my job, I am frequently asked whether a process is a good candidate for automation, and really from my perspective, the only logical place to start answering that question is with data. One of my favorite quotes is by W. Edwards Deming, he was an American engineer. He had a profound impact on Japanese manufacturing back in the 1950s. The quote is, “Without data, you’re just another person with an opinion.”
Anybody who’s worked in a team or a group project knows that everybody and anybody has an opinion or a suggestion about the best way to get things done.
Then there’s the question: Whose opinion is the best? Is it possible to have a system to help us get to the best answer? How can we apply an objective measurement tool to a group of subjective opinions?
Ultimately, recommending an automation solution should be a data-driven process. If we start from a solid base of data, enforce the decision-making process to consider that data throughout the assessment period, it often makes it very clear if automation is a wise investment or not. What kind of data are we talking about here? Honestly, from my perspective, the more the better.
Now, I’m only half joking, but I’ve helped clients in a lot of different industries, aerospace, hydroponics and grain trailers. I’m not an expert in any of those industries; our clients and our customers are. So, part of the assessment process is what I like to call drinking from a fire hose. It usually involves learning more about the process of making things than I ever thought I’d learn, and really the goal there is to try to learn as much about a customer’s process and product as possible in order to identify the best potential applications for automation.
Developing a thorough automation response
This typically requires a facility visit, a few days keyed completely around data collection. It means collecting days of video, talking to everybody from production managers to maintenance technicians, operators and engineers. We pull in sales, IT, safety teams, we get our hands dirty, we touch the product, we go through the process ourselves. The main goal is to understand as completely as possible our customer’s perspective in order to give better support to our recommendations in the future.
The more thorough we are in the data collection stage, the less surprises we have later and the more confidence the customer or client has in the process. The main areas of data we’re looking at, obviously this is usually in a manufacturing space, so product is the number one piece of data that we’re out there looking for. What are they making? What’s the historical production data, the part mix, part drawings, 3D models, dimensions and weights?
We’re looking for anything and everything because we don’t know what’s going to necessarily be important later on down the line. The next thing we’re looking at is process. How’s the customer currently making their parts? This involves anything from process flow maps, work instructions, the paperwork and the data that flows through the process and the next area is equipment. What are the tools being used to make the parts?
If you look at the industries in general, there’s a lot of custom machinery and tools out there. We’re looking for spec sheets, drawings and models. What are the preferred OEMs the customer or client prefers working with?
The process has to happen in a physical space. It’s important to know our sandbox. What is the facility and layout information? Is our space constrained at all? Is the customer willing to break new ground? Is it a greenfield? Are we ripping and replacing equipment or moving equipment around? A lot of the information we’re receiving in this category is required in order to pinpoint potential facility modifications. Do we need to pour new concrete slabs to handle heavier machinery or equipment? Where are the columns at?
Another major category is labor. How many current operators are being used? What’s the shift schedule? What does the labor pool look like in the area the facility is located at? Is it union or non-union? What’s the goal when it comes to labor? Are we trying to replace skilled labor with unskilled labor? Are we trying to bridge a labor shortage gap? And what does the desired labor situation look like from the business perspective?
Data collection and the five D’s
This usually comes in the form of high-level goals or target setting, where we’re looking at it from an overall business perspective. What is the main goal of an automation project in the first place? Is the customer looking to increase throughput? Are they looking to increase quality, reduce labor? What are their return on assets (ROA), return on investment (ROI) and payback requirements? Is it a two-year, three-year payback? The important one when it comes to buying the automation is what are our capital expenditure limits?
The goals in this data collection phase are really to tease out what is important to the customer, and we want all the stakeholders to agree on what the goals, the targets, the KPIs are in order to gauge whether that automation is a success down the line.
While we’re at the facility, some of the potential candidates for automation can be easily uncovered. Typically, these are known as the low-hanging fruit, and sometimes it’s called the three D’s, four D’s, five D’s, any of the processes or tasks you’re looking at fall into one of these or more of these categories typically can make a good case for automation. We’re talking dull, dirty, dangerous, difficult and dear.
- A dull process is a process that’s repetitive or boring. Anybody that’s worked on an assembly line, perhaps that is doing the same task over and over again, an operator who is not engaged will frequently make mistakes and quality tends to suffer.
- Is the task dirty? Is the process or the environment hazardous? The things we’re talking about here, welding and painting. A lot of these tasks have been successfully replaced with automation.
- Dangerous tasks. If the task is going to potentially cause harm to a human operator. Heavy loads, sharp parts and environments that contain hazardous gases. These are great places for automation or potential applications for automation.
- Difficult. Is the process hard for a human operator to execute? We’re talking small parts or heavy parts, awkward parts, things that are hard to assemble. Tight spaces, excessive walking or energy required are great applications for automation again.
- Dear. Is the process or the part critical or is it expensive? Tight tolerances can usually be more repeatable using robotics or other automation, and also the cost of a botched part due to a human error, something a lot of companies are looking to avoid, so investing in automation seems to make sense for that particular D.
Next steps for data collection for automation
At this point, we’ve collected all the data. While we were on site, we identified potential low-hanging fruit for applications for automation. What do we do now? Now it’s time to start analyzing all that data we received.
Depending on the quality of data, the missing pieces of data you don’t have, this could definitely take some significant time and energy. It also takes some intuition and some experience to know what you’re looking for, so at this stage we’re taking our product data and we’re breaking out a product mix. We’re putting things into product or process families. We’re identifying rates and dimensions and weights. Really what we’re trying to do here is get a handle on how the product is made and could it be made or processed with automation?
At the same time, we’re collecting all of the requirements and parameters that are going to support our automation decisions further on in this process, so a simple example here is you need to know the dimensions or the weight of your product in order to know what conveyors or robots can handle that product.
That’s a very simple example, but we’re trying to create a framework that will allow us to make objective decisions and recommendations later, so we’re identifying some SKUs that may be problem parts, they may be more difficult to make, they may differ significantly from the rest of the products that that customer is making. Those are all going to speak to the automation solutions in the next step of brainstorming and concept design.
We’re creating process flow maps, identifying inefficiencies in the current process flow so that those don’t make it into the automation. All the steps in this data analysis stage should be with the goal of defining requirements, setting up the parameters, and creating a decision-making framework for the next steps in the assessment process. At this point, we can usually take a look at our product mix. We should have that data and we can what I like to call a reality check question.
How to decide when using automation is worth it
We have what I refer to as our own version of the Pareto Principle, and that’s a rule of thumb that usually only makes sense if 80% of the customer’s product mix can be captured and automated. Anything less than that requires a much healthier business case, and usually the remaining 20% or less can be pushed to some sort of manual or semi-automatic solution, something that can handle maybe a lower throughput or rate.
Traditionally, this meant a chaotic product mix or a high variation low volume product mix, we’d struggle to make a case for the automation for that. The complexity of the required automation would price out most customers. However, the math can often be much simpler than this, and usually starker.
The question isn’t whether automation makes a good business case. It’s more of an existential question is do we automate or do we die? There are a few reasons why a company would be asking themselves that question. Some clients we’ve come across are in what I’d call a labor desert, towns with a couple thousand people that don’t typically offer a constant pool of labor resources. If the task at hand falls into one of those five D’s, you’re going to find labor that’s not really willing to do that anymore.
Sometimes, a bigger player enters the market, there’s a big warehouse that moves in down the street and starts to siphon off the labor pool and suddenly you’re shorthanded. It can be hard to convince workers to work in one of those five D environments when they could potentially make more down the road at a desk job. Another common story is a small company suddenly finds its product in high demand.
Suddenly, the need to go from a mom-and-pop shop to a manufacturing powerhouse. So in order to make parts, or more parts, the natural solution is to hire more people. That’s not always possible, so automation is required to fill that gap. And lastly, if a close competitor has made that automation leap, sometimes the only solution is to do so yourself in order to keep up.
At this step, we’re kind of getting into the trying to figure out if we need to redesign the part prior to automating. At this point, we’re analyzing the process for inefficiencies. The main thing we don’t want to do is automate a bad process. A pretty simple math calculation here. Automating a bad process makes you more capable of making bad parts faster, so in order to avoid that, the best process we’ve found is a brainstorming and concept development phase. Brainstorming usually broken up into a number of rounds.
How to brainstorm about possible automation use
We have an “anything goes” round where anything and everything is recorded as a possible solution. No idea is a bad idea at this stage. Anything goes. Then we had our kind of an editing round where we remove the bad or infeasible solutions. This is usually dictated by the data you collected and analyzed in the previous stages.
Then you start to bring the customer into it. Those first initial rounds are internal, you try to keep it with the small group, then you bring the customer into it so they don’t see all your bad ideas. We have some collaborative brainstorming with the customer, whittle down the options to a select view and say, “These are the ones that we want to either investigate for research or enter the pricing phase.”
During these phases, we’re asking some pretty standard questions. What are all the possible ways to make the part or the assembly? What steps in the process can be automated and which should be automated? If it can’t be fully automated, is there a semi-automatic halfway-there solution? If there’s not a good automation case, can we give the operator some tools to make their job produce more quality parts, make it more ergonomically friendly or just push throughput up?
We’ve taken all our conceivable automation solutions, and we’ve whittled them down to solutions that fit within our framework. The next step is concept development. There’s a ton of tools you can use here depending on what the customer is comfortable with. We typically do 2D and 3D layouts. We place the equipment in the defined sandbox we were given during collection. How does it fit in the space? Did we miss something during data analysis or brainstorming that may cause potential issues now that we’re looking at it in a 3D model? We can investigate potential robotic solutions via robot reach studies or cycle time studies, but we can do discrete event analysis to make sure we’re hitting the required throughput. We’re wrapping everything around that data framework we had created through our data collection and analysis phase.
How “design for manufacturing” can help with automation
For certain projects, if a process or part design is required, it may make sense to do what’s called a design for manufacturing or a design for assembly. When manufacturing individual parts, a design for manufacturability (DFM) is going to basically be a deep dive into the design of that part, and how we could potentially redesign the part to make any resulting automation less complex. How would we make it easier for a robot to grasp? Can we change the material to make it a simpler process?
The data collection and data analysis are going to set up parameters for what those possible potential changes are and whether they’re going to be acceptable to the customer. When we’re dealing with a collection of parts and actually assembling them, we would do what’s called a design for assembly. Instead of changing the unique parts to make them easier to manufacture, we’d be adding things like tabs or things that make them easier to put together into an assembly.
We could rework tolerance requirements, tweak the shape of a part to make it easier for automation to assemble it. Just a couple of specific examples for redesigning the part or the assembly around the process or the automation that we’d be proposing. At this point, if we’re left with multiple potential solutions that all meet our data framework, requirements and parameters, how do we make a solution when we’re left with multiple possible solutions? The best idea is let the data take the lead.
Decision matrix to prioritize automation opportunities
Typically, we would put together a decision analysis matrix that puts each of the potential solutions against those prioritized goals and targets from our data collection visit that came directly from the customer, and then depending on what the customer told you is important, some of those solutions may naturally rise or sink below the others. What is important to the customers is going to usually be the deciding factor.
Sometimes that’s a business case. Other times, it’s something as simple as footprint requirements or labor requirements, but ultimately the goal should be to remove the emotions and the subjective feelings from that decision making process and make it as objective as possible.
Typically, what we would do for any of our solutions is a business case, and the simplest way to do that is calculating the return on investment or the payback. If that doesn’t come from the customer, we typically would wrap that around a throughput increase or a labor reduction.
The main thing is machines are starting to catch up to humans. Artificial intelligence and machine learning are starting to optimize things like machine vision, random part handling, data monitoring, predictive maintenance, plug-and-play user interfaces and technologies that are making it easier for non-programmers to program. These are all areas that are starting to move in from that R&D, research and development sphere into proven and in production solutions.
Edited by Chris Vavra, web content manager, CFE Media and Technology, [email protected].
ONLINE
https://www.controleng.com/webcasts/best-practices-for-effective-automation-applications/