Digitalization applies software solutions that use data to improve or transform business processes; digital transformation can include many digitalization projects
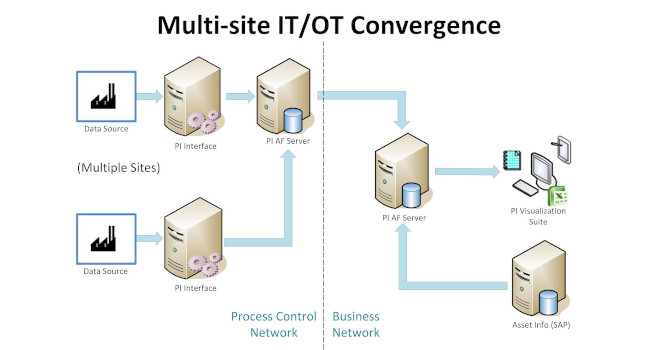
In the world of technical buzzwords, digitalization ranks at the top of the list of those most misunderstood. It is frequently misused and confused with similar terms such as digitization and digital transformation, as well as data acquisition, Big Data, internet of things (IoT) and Industry 4.0. If you are looking for a way to overcome a bad case of insomnia, I recommend that you search the internet for scholarly discussions that compare and contrast the nuances between the terms.
“Digitization” converts information — in our case process measurements and signals — into digital values. “Data acquisition” gathers, organizes and stores this digitized information in large quantities, typically using process historians, and more frequently storing this data in the cloud. (For an excellent article on data acquisition, refer to the article “Eight data acquisition best practices” in the December 2019 issue of Applied Automation.) “Digitalization” is the application of software solutions that use this data to improve or transform business processes. “Digital transformation” is strategic business transformation that includes many digitalization projects.
Digitalization solution examples
For those who have not yet been involved in a digitalization effort, it may be difficult to envision how it might be applied at your, or your customers’ facilities. This article will provide overviews of application examples of software tools that put plant floor data to work improving efficiencies, reducing energy consumption, preventing costly equipment failures and reducing the burden of control system engineers by moving the responsibility of developing and maintaining complex calculations into the hands of the end users of the data.
Control loop performance monitoring: Through the application of advanced analytics, PlantESP from Control Station simplifies both the identification of proportional-integral-derivative (PID) controller performance issues and the implementation of issue-specific corrective actions. It is a plant-wide monitoring solution that uses existing process data to proactively identify and characterize issues that negatively affect production quality and throughput. Common issues identified by this and other control loop performance monitoring (CLPM) tools include mechanical, reoccurring loop interaction and PID controller tuning. It allows plant staff to identify subtle trends and degradations in performance undetectable by the human eye.
An example of CLPM tools being used to identify the root-cause of a complex performance issue is the implementation of PlantESP at an oil extraction facility in Canada where plant engineers were unable to identify the source of variability within a critical production unit. A significant uptick in oscillatory behavior was undermining the unit’s steam quality, which resulted in reduced throughput. Upon implementation, this system immediately confirmed the behavior and quantified increased oscillations across multiple control loops. Using power spectral analysis, engineers quickly identified loops that shared the same frequency characteristics and they narrowed the scope of their search. With the help of the technology’s cross-correlation tool, they established the lead-lag relationships between each of the loops and pinpointed the root-cause: a valve with excessive stiction (“stiction” is the force required to cause one body in contact with another to begin to move). In a matter of hours, this software provided the answer that had eluded plant staff for weeks.
Asset performance management: ASSET360 monitoring and diagnostics product from Atonix Digital collects existing data from across the infrastructure, applies machine learning to alert personnel of abnormal behavior and streamlines problem diagnosis. It combines industry domain expertise with machine learning and advanced pattern recognition to identify early performance degradation of process equipment, allowing for maintenance of equipment before costly failures occur. Analytics are performed in the cloud and remote system monitoring services are typically coupled with the software (see Figure 1).
This system has been in service at a midwestern coal plant for the past three years. The ability to monitor, analyze, diagnose and resolve issues quickly and easily has greatly improved reliability, and has given engineering teams new flexibility to work on more strategic projects and initiatives. Early remediation also has extended the useful life of numerous assets. In the first three years after deploying the solution, the plant enjoyed cost savings as well as increased reliability from the early identification and resolution of more than 320 issues. Through a combination of extended asset life, reduced outages and other efficiencies, the plant has benefited from hundreds of thousands of dollars in savings.
Edge computing: Cloud based monitoring may be fine for identifying small changes in large pieces of process equipment over time, but not for immediate responses for high-speed equipment. An example of this technology is The Lightning edge computing system from Foghorn. It allows machine learning and analytics to be applied to high volumes of data at high speeds to recommend real-time equipment setting adjustments. Key pieces of the overall data being processed are typically sent to long term data storage systems such as historians.
At a large consumer products manufacturer, McEnery Automation worked as part of a project team to implement Foghorn’s edge technology to improve a high-speed packing line performance by monitoring package weights, recognizing patterns of variations and recommending equipment adjustments.
Multisite IT/OT convergence: Allowing data traditionally stored on the operational technologies (OT) side of the business (the controls network) to be shared with the information technology (IT) side (the business network) is a major transformation.
For a large water utility, historical process data was maintained in multiple SQL Server databases at various sites throughout the state, and was accessible only on the controls network. All regulatory and operational reports required by the business were managed by the automation and controls group. McEnery Automation assisted with migrating this data into an OSIsoft PI System. Local database interfaces buffered the data at each site before being sent to one central repository located on the controls network at the company’s headquarters facility. One PI Asset Framework (AF) server located on the business network functioned as the access point for the business network while organizing and contextualizing the data. This pair of servers created its own firewall between the two networks. A SQL Server database fed asset information to the AF server for use in contextualization. By organizing the data into standardized hierarchies in and adding contextualized data such as scaling in range and engineering units, equipment size, model and serial number, the data became much easier for business users to access and understand.
This new model provided benefits in multiple ways. By giving data access to end users, report generation was streamlined, and consistency was increased among sites. Removing the responsibility for support of the reports and the reporting servers allowed controls engineers to spend more time ensuring the quality and accuracy of the data going into the historian. Being able to compare process and operational data between sites identified opportunities to increase efficiencies and consistency. The engineering department was able to access data for use in comprehensive planning studies, transient pressure studies and predictive maintenance for pumps (see Figure 2).
One platform supports multiple applications
McEnery Automation has been a partner in the digital transformation of a global food and beverage manufacturer, assisting in the selection and implementation of an operational intelligence system infrastructure, as well as developing pilot implementations for multiple digitalization efforts.
Rockwell’s FactoryTalk Historian was used in all North American plants to collect data from various areas of the plants including processing, packaging and utilities. OSIsoft’s PI platform Asset Framework organized and contextualized data, making it more accessible to individual users. Tags were organized into multiple hierarchies based on user needs. For example, utilities department technicians may want to group data based on the systems such as hot water, steam and compressed air. Packaging technicians may want to group systems by packing lines. Metadata was added to provide context and descriptive information about the tags. In most applications, OSIsoft software tools PI DataLink, PI Asset Analytics and PI Vision were used to access the data, perform reporting calculations and present the information.
The intent of this system platform is to be a common data source for many applications throughout the entire company, both on the OT and IT sides of the business. McEnery Automation has assisted in the implementation of pilot applications varying from equipment maintenance, production scheduling, utilities monitoring, energy efficiency improvements and packaging line performance. Here is an overview of applications using data from the same platform.
Utilities monitoring: A legacy reporting system that monitored utilities department processes to minimize costs was replaced by aggregating and organizing real-time data from the utilities department into the process historian. Key process data included electrical power, fuel, CO2, compressed air, steam and water usage.
The benefits of this application included:
- It allowed utilities data to be available to other departments and to be correlated to production levels.
- Moving calculations from the PLC to analytics allowed process owners to manage calculations, which made the reporting process more efficient and reduced the workload for controls engineers.
- Allowed monitoring of key performance indicators (KPIs) over multiple sites and site to site comparisons.
Process department scheduling: A legacy production planning system was replaced by aggregating work-in-progress (WIP) process data from more than 300 process units and storage vessels into the process historian. This information data was made available for use by third-party supply chain production planning software.
The benefits of this application included:
- It allowed easy standardization and deployment across all process equipment.
- Complex manual calculations required of the existing system were eliminated.
- System maintenance and support were drastically reduced.
Packing line dashboards: A new application was developed to display key packing line information on one overview screen. Data from various sources (PLCs, OPC servers) for multiple packing lines was aggregated and organized into the common historian platform. The system provided access to packing line data using an internet browser and allowed drill-down access to packing equipment information such as equipment states, production counts, line efficiency and inspection/quality data.
Benefits realized:
- It eliminated silos of packing data by displaying information from multiple lines and multiple data systems on one real-time dashboard.
- An internet browser provided easy access on both OT and IT networks.
Packing line predictive maintenance: A new application was created to notify the maintenance department of early performance degradation of packing line motors. Data was collected for temperature and vibration from servos, variable frequency drives (VFDs) and sensors mounted on the motors. Equipment templates and hierarchies allowed easy standardization and organization of device alarm limits. E-mail notifications of alarms were sent to maintenance personnel for maintenance scheduling before the motors failed and caused production shutdowns.
Benefits realized:
- Cost savings were gained by scheduling maintenance before costly downtime failures occurred.
- One common system was used to collect data from multiple vendor devices.
Packing line energy consumption: A new application was developed to support an energy conservation initiative. Line status, motor running status and motor nameplate information was used to calculate energy savings gained when motors were properly stopped or slowed during line stop situations, as well as identifying potential energy savings from motors that remain running during line shut downs.
Benefits realized:
- Energy savings from ongoing improvements in equipment operation due to awareness of potential improvements.
- Use of existing motor metadata and status simplified implementation.
- Use of specialized analytical tools outside of the PLC and human-machine interface (HMI) programs simplified implementation and removed responsibility of programming and support from controls engineering group.
In conclusion
In each of these examples, a business process was transformed or streamlined in some way, whether by applying advanced analytics, giving access to the data to the end user, aggregating data from multiple sources and sites or moving complex calculations out of the control level systems. In most of the examples, data already being collected was able to be used for a new application. This supports the principle that getting a robust process historian in place and collecting as much data as reasonably possible is the first and most critical step in digital transformation. It is imperative for any manufacturing company that wants to remain competitive in the evolving business climate.
Once the infrastructure is in place and data is available, the ways in which the data can support digitalization applications are many. Some are simple and straightforward. Some are creative. Most are proactive instead of being reactive. They allow employees to reduce the amount of time supporting complex systems and reacting to equipment failures and increase time spent proactively developing process improvements with the new technology available to them. And after your organization has a few of these under their belt, who knows, you just might be in the middle of a digital transformation.
This article appears in the Applied Automation supplement for Control Engineering and Plant Engineering.