After a webcast on “Advanced process control: Past, present and future,” more answers to audience questions are provided by the webcast speaker covering advanced process control (APC) to help with process optimization.
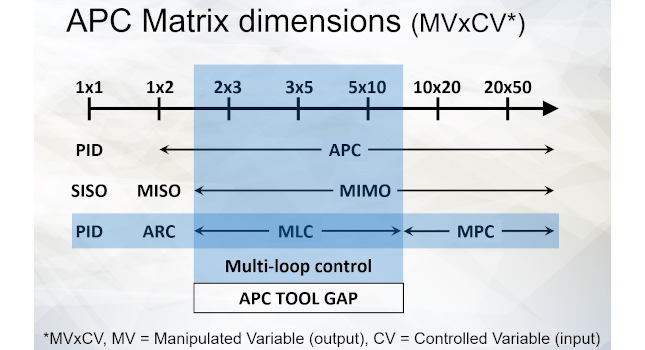
Advanced process control (APC) comprises a number of tools and techniques whose common characteristic is taking process automation beyond the limits of single-loop control and into the realm of multi-loop (or multivariable) control. The Jan. 9 webcast, “Advanced process control: Past, present and future,” aims to help process engineers (also known as production or operation engineers) increase understanding of how modern control systems can improve process performance and optimization.
At the end of the webcast, registrants viewing live ask questions. Below are additional questions and answers the webcast didn’t have time to address. If there are any additional questions for Kern, contact him here.
Question: Please explain more about what you meant when you said MPC has invested a lot of unplanned resources.
Answer: Model-predictive control (MPC) has invested a lot of resources over the last several decades, both users and suppliers. The question is whether that has been done in a planned or unplanned way. The industry should have evolved towards multi-loop control (MLC) — more agile, lower cost, easier to use tools.
But instead, most effort has been struggling with model quality, optimizers, and matrix design. The struggle continues and the cost and complexity of ownership has not come down; if anything, cost and complexity have increased.
Industry has learned why these issues won’t be solved (in the case of modeling), or why maybe they are not worth solving at this point (in the case of optimization).
Industry should move on to more manageable and promising APC 2.0 solutions with the benefit of this experience.
Q: How can the “mighty matrix” be more significant in APC than models can?
A: This is a good question; the matrix is a relatively simple concept, while model-based control is a very advanced control concept, so logically counts for more.
But the point is, like a single-loop trend vs. the proportional-integral-derivative (PID) algorithm itself, the matrix is a simple tool that allows better visualization and understanding of multivariable control, and ultimately that can be more impactful than the nitty-gritty of the algorithm itself, if that makes sense.
Also, industry is realizing more ways to do multivariable control beyond MPC. The matrix is a basic underlying tool, regardless of how it’s carried out — manually, MLC, or MPC.
Q: I have seen papers online under both APC Performance and Lin & Associates in your name. What is the difference?
A: APC Performance is my control consulting business, but I also work closely with Lin & Associates (L&A), based in Phoenix. L&A is a well-known medium-sized control system integrator in Phoenix, for more than 25 years, with many long-term Fortune 500 clients in oil and most other industries. In addition to L&A’s traditional business, I am partnering with L&A to develop APC 2.0 tools for industry.
Q: What industries other than HPI do you have experience? What is the longest in-service application you have? How prominent are services in long-term APC support?
A: The MLC tools mentioned are available already, with some applications having been online several years. The controllers have run essentially 100% uptime and maintenance free.
I have worked in hydrocarbon processing industry (HPI), chemicals, and power. L&A, the system integration firm mentioned above, has experience in virtually all industries: oil, gas, chemicals, power, paper and some commodities.
Q: Does an APC always guarantee plant running at optimal conditions? Does the multi-loop ensure this?
A: APC guarantees the plant is running at optimal conditions, if:
- The APC application itself is running.
- It is not wrongly constrained or defeated by pinched limits, clamped manipulated variables (MVs), etc.
- It has the correct matrix.
- MPC complexity and fragility have worked against achieving these, as has the large matrix design paradigm.
- A goal of MLC is to transcend these “fragile” sources of breakage.
Q: What about benefit estimation, performance monitoring and maintenance?
A: The first and often most important benefit is the intrinsic benefit of closing loops. Everyone in process control understands this is the goal. In many applications, this is sufficient justification, especially if cost is low (MPC cost is high, so greater justification has been needed).
In MLC, just like MPC, in some applications, benefits can be quite high. I’m working an application now that is estimated to be worth several hundred thousand dollars annually. In APC 1.0, it’s been well known that a large part of the benefits often come from just a few variables or just a few models, not the entire matrix. Performance monitoring is an accidental sideline — one of the unplanned activities, and essentially impossible, as I mentioned under performance criteria — error minimization vs. safe driving habits. Under MLC, I see performance monitoring, as we’ve come to think of it under MPC, going away.
Q: What about APC creates value?
A: The obvious and intrinsic benefits of closing loops provide value and simple, reliable MLC control. That’s important.
Q: Regarding adaptive gain, when should non-linear gain be used?
A: First, I would emphasize using cascade where possible. Cascade and adaptive gain are complementary. Cascade linearizes gain, instead of using adaptive gain.
Beyond that, honestly, I’m not a big fan of adaptive gain in practice. It is useful as a learning tool, to illustrate how control algorithms work, but is often troublesome in practice. Like unnecessary use of feedforward, adaptive gain requires assuring the model matches the process, and if not, performance may be made worse for all your trouble.
Nor am I a big fan of gap gain in level control, something which also gets more attention than it warrants, in my view. See “Top 10 tuning tips for control engineers.”
In most cases, I would rather use an average gain (or the minimum gain, as the application dictates) and see if I can live with that.
Q: When talking about APC, where do split-range control, ratio control, and the Smith predictor fit in?
A: I would place these in the category of advanced regulatory control (ARC). These are all three important tools to have in your box, along with feedforward and override. And maybe also adaptive gain or cascade.
Q: What is the operating envelope?
A: Slides 20 and 21 in the webcast show a pentagon representing the operating envelope. The circle is the minute-to-minute operating region, while the pentagon is the overall envelope (or window). The two-step goal of APC is to put the operating region within the envelope, and then move it in a direction of greater optimization to the extent it can.
Q: Aren’t models still needed (lag time, process gain and dead time) for controller tuning?
A: Yes. As a feedback controller, MLC (or my version, model-less multivariable control called XMC), will have some basic tuning needs, but also latitude in reliable values, and with a conservative or safe direction to err on the side of stable performance. It’s similar to PID in this regard, but is unlike MPC, which depends on model accuracy.
Q: How would you know when to go for MPC, and not end up with a bunch of MLC solutions?
A: Appropriate MPC applications are well established by now. Use the technique shown in the presentation to identify MLC applications. In most cases, the applications will be operationally problematic, in terms of creating numerous operator interventions and alarms, i.e. most applications will “find you”, once you realize how to spot them.
Q: What programming languages are required to design a non-in-built distributed control system (DCS) MPC?
A: A custom reusable function block, now available in most modern DCSs and programmable logic controllers (PLCs), is the target deployment mechanism, although with today’s flexible technology, other options present themselves. Visual Basic (VB) or C++ is good.
Q: What platforms do you expect will support MLC? Embedded controllers or servers, or what?
A: DCS control layer and (with more limits) PLCs can support MLC. In some cases, users may prefer a generic network-based solution, but this adds back complexity and other issues for the owner/operator.
Q: Should an MPC require continuous data for the step/pre-testing?
A: Theoretically not necessary, but in a continuous run, there is more continuity and ability to spot potential plant events that may cause data irregularities.
Q: Not enough emphasis was given to the need that we engineers KNOW our processes inside and out.
A: I agree completely. In MLC, the matrix is designed based on existing (or desired) manual multivariable control methods, that is, it is based on automating the way the operating team already knows how to manage constraints and optimize variables.
Q: A major process industry software provider… now offers online dynamic model updating in a couple of forms. Are you familiar with this and have you seen any related applications?
A: I am familiar with the software you mention, but have not used it. This represents the auto-tuning story all over again, which is one of the big past lessons that I did not have time to address in the presentation. Auto-tuning can be looked at as an attempt to create an “adaptive model” for a single loop. For the most part, industry failed at this, despite a lot of trying. So, how likely is it to succeed on a multivariable basis that is geometrically bigger and more complex? This adds yet another layer of cost and complexity to MPC, with, in my view, doubtful prospects. I wrote about this in a related Control Engineering article: “Pros and cons of autotuning—the big story.”
Q: Moving towards a more feedback-centric (as opposed to model-based) paradigm, will MLC be able to respect constraints/limits as well as MPC currently does?
A: I believe so. For example, a simple override is actually a multivariable constraint control and optimization tool: The normal setpoint is the optimization target; the override setpoint is the constraint limit; the selector is a 1×2 matrix; and below the selector is the MV. We know these overrides are absolutely reliable and do not use models or optimizers. These well-performing ARC configurations exist throughout the industry and nobody ever considers that they may need or benefit from a model (although technically, if you were to do it with MPC, there would be a model between the MV and constraint variables). But we know that is not necessary. I think the industry will find the feedback MLC will solve many multi-loop problems simply and reliably.
Q: Do you have data that indicates the longer-term performance problems and maintenance requirements of MPC? Can you share this data? You made some very solid points, and some data to back it up would be good.
A: Nobody has or publicizes such data, but, slowly, over the years, the story has emerged, and is now generally accepted, even if people still don’t talk about it very openly. A high-available MPC with high utilization is rare; low availability and low utilization are more often the rule than the exception. I have been publishing on this topic since 2005. At some point, I discerned the root problems — short-lived models, the mistaken assumption of error-minimization performance criteria, and oversized matrix designs that exacerbate the first two root issues. At that point, I began suggesting changes, rather than fixes, which has led me to MLC.
Q: How about using closed-loop model-identification techniques in the maintenance of MLC or MPC?
A: I am not a fan of detailed model-identification in general. The majority of models add maintenance and risk while costing money and time. Wherever possible, use feedback. You will know where a feedforward model is needed, because it will be kicking you in the tail operationally. In most of the limited number of cases where a feedforward model is truly needed, it already exists in the base layer controls. I am especially doubtful about “adaptive modeling.” See my prior answer on this topic and related article link.
Q: Are you familiar with Cooper, C.D., Alley, F.C. and Overcamp, T.J.; (1982); Environmental Progress; 1; 129?
This is a model-based analysis method that can be adapted to MPC. It is generic to a single process — thermal oxidation of VOCs — with the parameters different for different VOCs. This is clearly a form of MPC that would work well in your middle-range of four independent variables and one dependent variable. I don’t think many thermal oxidizer owners are using MPC, but I think they might want to try it as a means of optimizing quality (i.e., high destruction) while minimizing fuel consumption (i.e., low operating temperature). See also Martin, R.J.; (2019); “Advanced Quality Methods for Thermal Oxidizer Operation”; Paper #71EA-0175; presented at 11th U. S. National Combustion Meeting; Pasadena, California, March 24-27, 2019.
A: I am afraid I am not familiar with the method mentioned, but here are my thoughts based on the excellent question. I think there are many high-speed multi-loop applications out there in the middle matrix range that have not been served by MPC, since MPC is expensive for mid-range matrix designs and (more to the point) cannot execute at 1-second, as MLC can. This appears to be one of those applications, based on your description. Moreover, the faster loops are, the less model-based control is needed, and the more effective feedback can be. I would like to consider one of these applications using our MLC tools.
Q: Doesn’t performance depend on the definition of the error?
A: I agree. Please see my answer (below) regarding the mistaken assumption of error-minimization performance criteria, which is also described in the webcast slide 24.
Q: What about dynamics, process changes?
A: Many or most of the dynamic questions, such as inverse-response, become less important or troublesome when feedback is used. Inverse-response is mainly a problem that came out of model-based control (you never heard of it before then). Model-based control first high-lighted the ability to handle inverse response, then turned around and provided detuning knobs to ignore fast dynamics! Similarly, model-based control is theoretically good for deadtime, but cannot handle not variable deadtime, which most applications are (based on feed rate changes). Deadtime always needs to be considered, even in feedback. (See the article on Control Engineering “What is rate-predictive control?”)
The following questions were verbally answered in the webcast, but the answers provided below offer more detail.
Q: Please clarify how MLC would do optimization without optimizers?
A: First, as on slide 23, optimization occurs at business level, where all the needed tools and information are available.
At the control layer, or APC layer, optimization refers to how excess MV availability, or degrees of freedom, is used. (It’s a two-step objective; refer to slide.)
So the question becomes: Where do the APC optimization targets come from — Production Planning & Optimization (PP&O), engineers and operators, and sometimes live calculations. They really can’t come from control-layer optimization, because there is no information available there outside of the controller itself. By the way, most targets rarely change; a limited number may change daily, and that comes down in the PP&O plan.
Unfortunately, optimization is a critical aspect that has been poorly understood throughout the APC 1.0 era.
Q: I don’t follow how error-minimization is a mistaken assumption? (If not error minimization, what?)
A: If you’ve been a control engineer a long time, you can see a pattern… sites embark on tuning/modeling campaigns, followed by periods of detuning or move suppressing.
The problem is, error-minimization is an aggressive criterion leading to fast/large MV movement, and excessive overshoot and oscillation.
In industrial process operation, these are the least desirable performance characteristics. It is much more important to get to setpoint at safe speed and with minimum overshoot or oscillation.
Unfortunately, this (latter) type of performance does not lend itself to easy or mathematical measurement, but can only be gleaned by overall metrics, such as loops in manual, multivariable loops in manual, number of alarms, etc.
Q: There are many questions about artificial intelligence (AI), deep learning, Industrial Internet of Things (IIoT), recovery time objective (RTO), etc. What are their implications for APC?
A: AI is an exciting field, but it’s not really my field, and I don’t see it having a big impact on APC within my (now somewhat limited) time horizon.
I see AI as a tool to do things faster, but not necessarily better. If modeling cannot solve the problem with all the effort we have seen, then I don’t think AI is going to work, until we understand the nature of the problem.
Somewhat surprisingly, even to those of us in the business, most plants are highly variable, not the same week to week — many variables change, and there seems to be an endless supply of new types of disturbances.
Industry saw this in benchmarking; we’ve seen it in APC modeling; and we’ve seen it in neural networks for inferentials.
So, my hat is off to the AI crowd, but it’s not too big on my radar at this time. At least not for APC.
Q: How does model-less APC work?
A: Model-less can basically be thought of as feedback, whereas model-based is basically feedforward.
That said, the algorithms used in our MLC tool (XMC) have been patented. They represent the industry’s only “inherently adaptive” control algorithm, and the only “model-less” method of multivariable control. And they are uniquely well suited to multi-loop applications.
Q: Fuzzy logic (FL) does work when applied correctly. I have direct experience with that. The key is having a solid understanding of the process and appropriate application of FL.
A: Thanks for that feedback. As I say, fuzzy logic always comes up, so there must be some satisfied users out there. In continuous APC, we normally do not need or avoid any kind of discontinuous breakpoints. That’s one reason I don’t even like gap level control (although I have additional reasons for that as well).
Q: Please discuss fuzzy logic and feed-forward control.
A: Fuzzy logic always comes up in the discussion, but I have never used it.
Feedforward, on the other hand, is a very important topic — every control engineer should know it; it’s not complicated. Feedforward is the single-loop equivalent of model-based control. It’s an essential or critical technique for some applications, but only on a very selective basis. It’s not meant to be used in a shotgun way as it has been in used in APC 1.0.
As mentioned, you don’t need to worry about missing a feedforward model — it will find you. Deadtime control is also critical; learn to use smith predictor with variable deadtime.
These topics may be covered in more detail in a future webcast.
Allan Kern, P.E., is owner, APC Performance LLC. Edited by Mark T. Hoske, content manager, Control Engineering, CFE Media, [email protected].
KEYWORDS: Advanced process control (APC), multiloop control (MLC), matrix
More answers after webcast on past present and future of APC
Process engineers can use APC to improve operations.
A matrix, used with APC, allows better visualization and understanding of multivariable processes.
CONSIDER THIS
Are your multivariable control applications running open loop? How much optimization are you missing?